TensorFlow を使ってみる
TensorFlow を使用すると、あらゆる環境で実行できる ML モデルを簡単に作成できます。 インタラクティブなコードサンプルを使用して、直感的な API の使用方法を学習しましょう。
import tensorflow as tf mnist = tf.keras.datasets.mnist (x_train, y_train),(x_test, y_test) = mnist.load_data() x_train, x_test = x_train / 255.0, x_test / 255.0 model = tf.keras.models.Sequential([ tf.keras.layers.Flatten(input_shape=(28, 28)), tf.keras.layers.Dense(128, activation='relu'), tf.keras.layers.Dropout(0.2), tf.keras.layers.Dense(10, activation='softmax') ]) model.compile(optimizer='adam', loss='sparse_categorical_crossentropy', metrics=['accuracy']) model.fit(x_train, y_train, epochs=5) model.evaluate(x_test, y_test)
ML を使用して現実世界の困難な問題を解決する
TensorFlow を使用して研究を推進し、AI を活用したアプリケーションを構築する例をご覧ください。
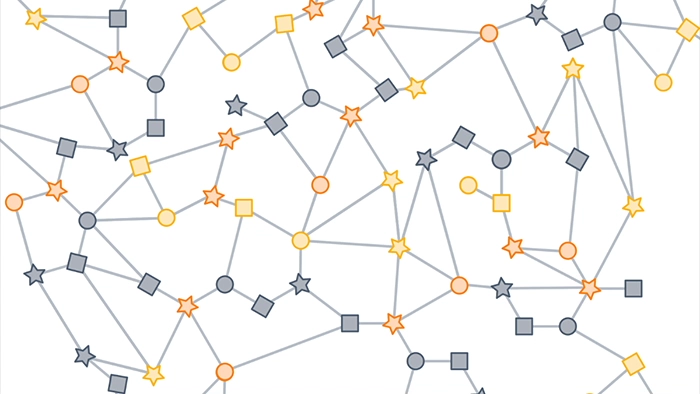
GNNs can process complex relationships between objects, making them a powerful technique for traffic forecasting, medical discovery, and more.
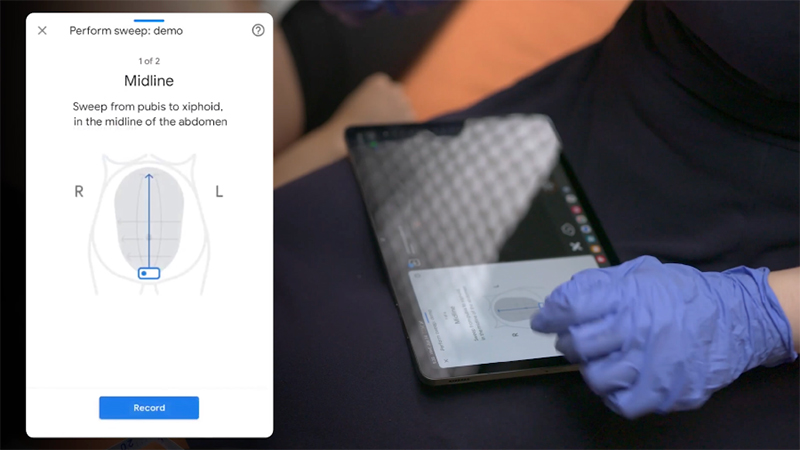
TensorFlow Lite を使用して胎児の超音波診断へのアクセスを可能にし、ケニアをはじめとする世界中の女性や家族の健康状態を改善している方法をご覧ください。
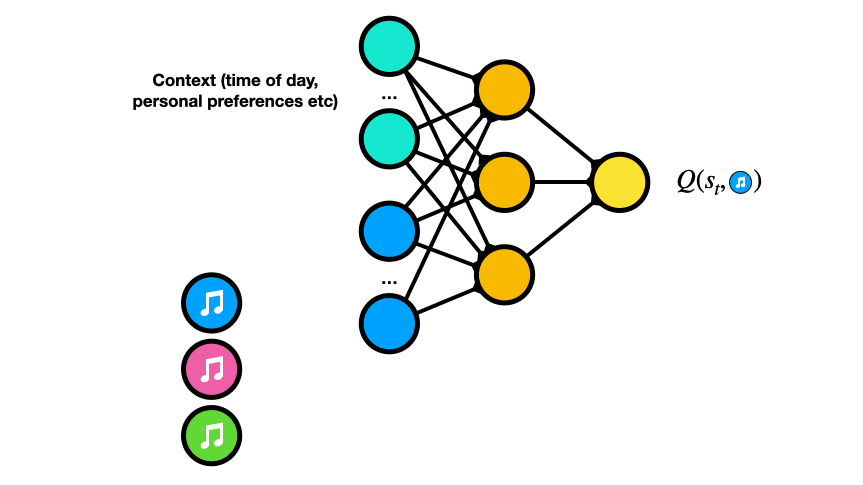
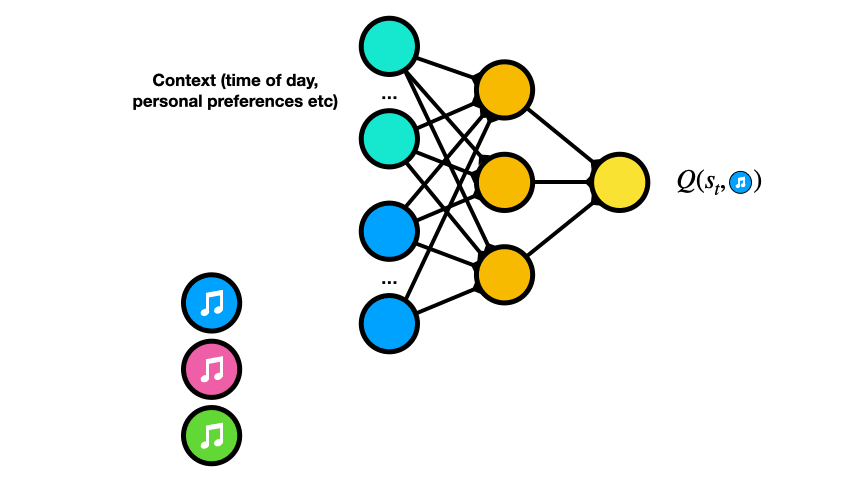
Spotify が TensorFlow エコシステムを活用して拡張可能なオフライン シミュレータを設計し、RL エージェントをトレーニングしてプレイリストを生成した方法をご覧ください。
TensorFlow の新機能
TensorFlow チームとコミュニティからの最新のお知らせをご覧ください。
エコシステムを詳しく見る
モデリングやデプロイなどのワークフローを加速させる、本番環境でテスト済みのツールをご確認ください。
-
Library
TensorFlow Lite
Deploy ML on mobile and edge devices such as Android, iOS, Raspberry Pi, and Edge TPU.
-
Library
TensorFlow.js
Train and run models directly in the browser using JavaScript or Node.js.
-
API
tf.data
Preprocess data and create input pipelines for ML models.
-
Library
TFX
Create production ML pipelines and implement MLOps best practices.
-
API
tf.keras
Create ML models with TensorFlow's high-level API.
-
Resource
Kaggle Models
Find pre-trained models ready for fine-tuning and deployment.
-
Resource
TensorFlow Datasets
Browse the collection of standard datasets for initial training and validation.
-
Tool
TensorBoard
Visualize and track development of ML models.